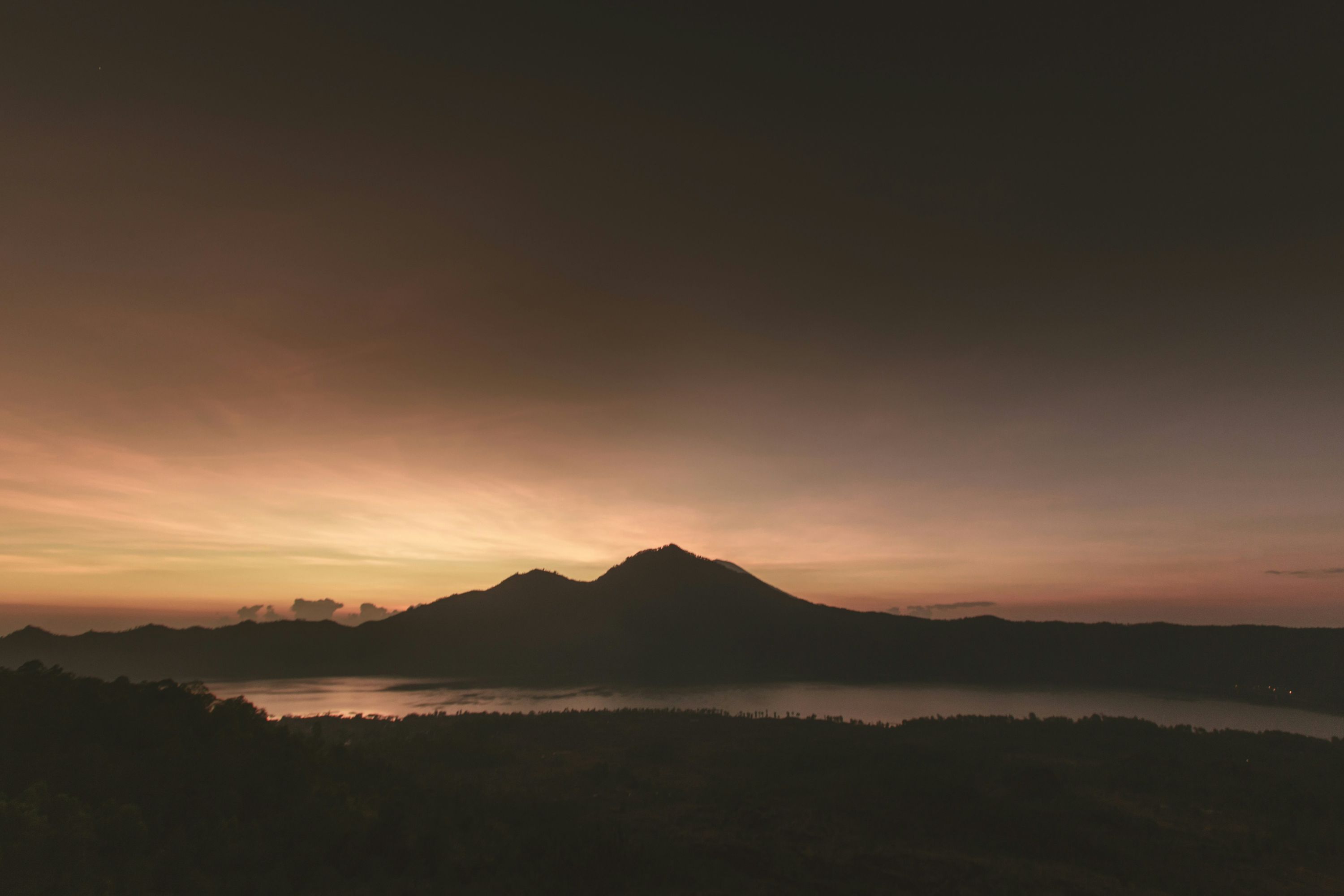
Theorem Overview
- The Skorokhod Embedding Theorem allows embedding of a random variable with zero mean and finite variance into a Brownian motion at some time t
- It demonstrates that any such random variable's distribution can be found locally in a Brownian motion
Simplified Explanation
- Brownian motion can be described as a random walk with unpredictable up or down steps
- The theorem guarantees that at certain points in time, the Brownian motion's distribution matches that of specific random variables
Applications and Usefulness
- Useful for simulating asset behavior and estimating future prices in financial modeling
- Can provide probabilistic solutions to certain partial differential equations, such as the heat equation
- Bridges discrete random variables and continuous-time stochastic processes
About the Mathematician
- Anatoli Skorokhod was a Ukrainian mathematician who contributed significantly to probability theory and stochastic processes
regarding yesterday's discussion
about theorems uh let's first talk about uh socro horde embedding theorem and let's talk what part so basically this theorem as it's the name is it gives us a way to embed a any random variable distribution like we choose a distribution or sorry we choose a random variable with fix mu which is zero and the sigma to be finite and now this random variable with this parameters or like with this conditions that mu is the zero and finite variance we will have a distribution of x let's say this random variable is x now on the other hand we have a Brownian motion. What this embedding theorem says is like the locally at some point the Brownian motion has the same distribution as this random variable with zero mu and finite variance. And this theorem only talks about the existence of some time t. And it's like, and if we talk about the word embedding, it means it simply says that any random variable with a distribution and mean zero finite various can be embedded into a Brownian motion for some time t.
now as an explanation
of this statement to a 14 year old which may not be able to understand what a random variable is what distribution mean what the brownian motion is so we can nicely translate this idea and then hence the theorem first we need to translate these terminologies that we can simply say what is this brownian motion so brownian motion for a 14 year old simply can be said like it is a random walk where one moves forward or backward but that steps are taken randomly and the path that someone is taking is not the straight path so it goes up or it goes down and once again it is unpredictable so we do not have the predictability that it goes up or down. So this is what a Brownian motion is and then we simply use it as like the time step at each step we are taking so we are counting as this is the one step at a time. in this particular manner is the target destination let's say we have some destination when we are walking but this destination does not mean it is like the end so in this theorem like when we are saying there is one x let's say along the we have one variable random variable with the distribution and that is an x we have another random variable y with another maybe distribution and then we have z so every time when we are moving along the path we are having a random walk we will have a point some point t is equal to let's say a where this random walk has the same distribution as x and then there might come not might come this theorem says there sure for sure come some other time t that could be before this x or after this and such that the distribution of our random walk which is a brownian motion is the same as the distribution of y So what we are doing, as I already said, so picking some random variable with the distribution and embedding locally into the Brownian motion. So at some point t, this Brownian motion has distribution as x. At some other point t is equal to b, this Brownian motion has a distribution as distribution of y, and so on. you
about good and bad part
let's talk about the bad part first so when i was explaining yesterday the bad part was like i was trying to explain my calculations like how i am picking each value in my google sheet and then i am sharing and what are the meaning of the numbers that i'm getting at the end of the calculation but of course for a 14 year old this may not be like understandable because i'm mainly just explaining calculation validation and generation part with the help of google sheet instead what i should have do is like verbally talk to the 14 year old explain in a story manner or something like what part is more important instead of how part i was trying to explain how this is working but i think what comes as at the very first step so that that was the like bad thing that I understand.
now the time is like good part the good part is i was able to touch the theorem feel it with the numbers like i can play around with it i can have a generator that can generate some results and then i can have a validator on the same time so first generating then validating and so basically it is like now i am i have that theorem in my hands i'm putting those things in the position it's like i'm playing a game now the theorems condition and statements are now my like the components on my chess board and then i'm putting them arranging it checking what what is like i tried even like a couple of distributions and things and i was thinking okay what is happening if i'm moving something here what does it change and then i can have a feel that what are the boundary of this theorem i can also check like what is the necessary why this condition to have to mean to be zero is a necessary because when i picked the mu to be a non-zero there was like no point i was able to find where that brownian motion have that particular distribution so something gets broken i was not able to fully grasp that something but i was able to understand that there is so this was the good part on my side that i was happy and enjoying touching and feeling the theorems with the help of numbers and then the good part of the explanation that i wrote was like when i was writing along the way there were several questions popping into my own mind as well so when i'm writing okay i'm writing let's say this brownian motion is a random walk and we move forward and backward on the same time my brain is processing these statements as well and bringing up some questions that i look on the google use chat gpt and i am learning more when i'm like writing or writing for someone else so that's that was a good part like i'm trying to explain to an audience and when i'm trying to explain my brain is behaving as an audience as well as as a like speaker so in both ways when this is happening i am enhancing my learning so that was a good part that i i i think it happened in this way
About the usefulness of this theorem,
I would say it is useful in a way that we can embed not any but like some distribution with a particular condition into a Brownian motion. So in our like let's say we are working with an asset and we have the Brownian motion of the path or like the time series that we have now by embedding different distributions into bronion motion we can simulate that particular assets behavior and we can also calculate the uh or estimate not calculate we can estimate the price at a certain time although we will not be able to so this theorem has some limitation will not be able to give us that exact particular time but it will guarantee that that specific price or the estimation of this value will happen in the future at some point so this is kind of useful in a way that it can be used for the prediction model that's one example second example what we can say is since this theorem also gives us some solution for the pdes so we can construct some not exact solution but probabilistic solutions and those not solution to all pd but the certain pds the reason is like why the specific pd is because the distribution that we are using has to have some conditions on it so we can have like a heat equation yes that that can be modeled in a way and then this theorem can provide a solution for that one
And another example,
sorry, you sent another question that checking this researcher, but I just looked at the images and found out like, let's say we are having a probability of something, an event happening. We can simply say we are flipping a coin and we want to model how much time we have to wait until a head will appear. But this will be simply not an exact amount of time but an estimation that can be modeled with this theorem as well. Now I will check this researcher's scoreboard and his other research. I will send you the voice note.
Looking at his Wikipedia and research papers, full name is Anatoli Sokrohod, and he's a Ukrainian mathematician, and he has like really good research work. First, our main his research field is probability theory. He has worked in like stochastic processes as well particularly. He has some results in stochastic differential equations and then there are limit theorems like about the convergence and those theorems and those theorems even have his names and then functional analysis and they have mathematical physics as well so these are the fields that his research is and the concept or like the specifically results that has his name is the most famous is so crow hod space even there is an integral with his name then this embedding theorem that we have been looking for there is one representation theorem as well i am interesting to look in this one but i will send you the detail in next voice note and yes he has around 450 scientific works this is a pretty decent amount of work with like significant mostly even mathematicians these days have thousands of research work but not that much significant and he has written some books as well
the purpose that could be redefined
of this theorem which is so cruel code so cro code embedding theorem is i would say providing us a bridge between uh let's say two stochastic frameworks or maybe more than two because this is not limited to two so what we are like getting out of this theorem is embedding a discrete random variable into a continuous process that's that's what like the main uh bridge is between the discrete and continuous time stochastic process so this theorem enables us to analyze uh complex probabilistic systems using a well-established theory of brownian motion because we have a lot of research already about a given about brownian motion and now we have let's say our discrete random variable because there is no particular condition on x except for the mean and variance this is still a limitation but not so we are limiting x but not very huge limitation with a certain we can say this is like with a certain condition not with the limitation and about checking uh something about his research later as i even sent in the previous first note i'm interested to look at this representation theorem which comes with his name i opened and see that what this does is that if we have a weekly convergent sequence of probability measures and the limit measure is sufficiently well behaved then it can be represented as distribution that's what the theorem statement of the theorem is but this is like interesting because the convergence then can be considered as pointwise convergence so once again we have a sequence which is like discrete but again the pointwise convergence sequence of random variable is again like the discrete uh just this one random variable but this can be defined as a on a common probability space so i think this this could be useful to look at it and then uh it's scientific work there is this limit theorem of random process as well which is of course the similar thing because in even in the representation theorem is talking about the limits and weak convergence so i think looking at these two concepts limit and convergence could be useful.